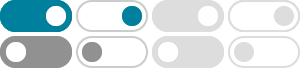
Simply Statistics
Code is a useful representation of a data analysis for the purposes of transparency and opennness. But code alone is often insufficient for evaluating the quality of a data analysis and for determining why certain outputs differ from what was expected.
Simply Statistics: Universities Do Spend Indirect Costs on Research ...
1 day ago · So this adds up to 20,000 square feet of space. The university can either build space or rent. Building 20,000 square feet of lab space can cost $25,000,000 - $75,000,000 and then the university has to maintain it, and upgrade every 15-25 years.
About this blog - Simply Statistics
We are three biostatistics professors (Jeff Leek, Roger Peng, and Rafa Irizarry) who are fired up about the new era where data are abundant and statisticians are scientists.The views represented here are our own and do not represent the views of Johns Hopkins University, Harvard University or Dana Farber Cancer Institute.
Divergent and Convergent Phases of Data Analysis - Simply …
There are often discussions within the data science community about which tools are best for doing data science. The most recent iteration of this discussion is the so-called “First Notebook War”, which is well-summarized by Yihui Xie in his blog post (it is a great read).. One thing that I have found missing from many discussions about tooling in …
Is Code the Best Way to Represent a Data Analysis? - Simply …
Jul 28, 2022 · Code is a useful representation of a data analysis for the purposes of transparency and opennness. But code alone is often insufficient for evaluating the quality of a data analysis and for determining why certain outputs differ from what was expected. Is there a better way to represent a data analysis that helps to resolve some of these questions?
Toward tidy analysis - Simply Statistics
May 23, 2017 · Tidy data at its heart is a set of three rules for organizing a data set:. Each variable forms a column. Each observation forms a row. Each type of observational unit forms a table. This is an incredibly useful abstraction for thinking about organizing data sets for analysis.
Simply Statistics: The analyst is a random variable
Jan 3, 2023 · I read this really interesting paper over the break, where they had multiple analyst teams analyze the same data set and fit a model to answer the same question.. This is a topic we’ve thought about a lot in the past; mostly from a therotical perspective. We have discussed the researcher degrees of freedom, recipe tradeoff and how p-values are just the tip of the iceberg for analyst variability.
The four eras of data - Simply Statistics
Dec 15, 2016 · I’m teaching a class in data science for our masters and PhD students here at Hopkins. I’ve been teaching a variation on this class since 2011 and over time I’ve introduced a number of new components to the class: high-dimensional data methods (2011), data manipulation and cleaning (2012), real, possibly not doable data analyses (2012,2013), peer reviews (2014), building swirl tutorials ...
Context Compatibility in Data Analysis - Simply Statistics
May 23, 2018 · All data arise within a particular context and often as a result of a specific question being asked. That is all well and good until we attempt to use that same data to answer a different question within a different context.When you match an existing dataset with a new question, you have to ask if the original context in which the data were collected is compatible with the new question and the ...
Four secrets of a successful data science experiment - Simply Statistics
Jun 3, 2016 · Editor’s note: This post is excerpted from the book Executive Data Science: A Guide to Training and Managing the Best Data Scientists, written by myself, Brian Caffo, and Jeff Leek.This particular section was written by Brian Caffo. Defining success is a crucial part of managing a data science experiment.